Homayon Afrabandpei
My role: Supervisor
Master Thesis Title:Mixture of probabilistic two-dimensional canonical correlation analysis, 2014
Abstract
From the time the first computers built, experts always tried to find out how to
improve the performance of computers in processing a large volume of information in
a way that acquire more accuracy by spending less time and resources. By the daily
growth of data and the noise inside them, experts concluded that data have to be
processed, too, before entering the algorithm as input. This is called pre-processing.
Nowadays, dimensionality reduction is one of the most important pre-processing
techniques used in different sciences. Feature extraction is a dimensionality reduction
technique and it is a collection of methods which try to reduce the dimension of data
by decreasing the number of effective features in the data. Feature extraction methods
are broadly divided into two groups: stochastic features extraction methods and
probabilistic feature extraction methods. In stochastic methods, only linear or nonlinear
transformations are used to derive a new feature space where the dimensionality
of data reduces when they mapped to this new space. On the other hand, Probabilistic
methods try to derive a new feature space by adding noise to the model and
considering a probabilistic distribution for each model parameters. Canonical
Correlation Analysis (CCA) is a well-known stochastic feature extraction method. In
this study we assessed different aspects of feature extraction; mainly the CCA method
and we proposed a new probabilistic model for CCA along with a mixture of
probabilistic CCA model. The proposed methods are evaluated in a face recognition
application and the results showed that using these techniques, the classification
accuracy improved well.
Keywords: dimensionality reduction, feature extraction, canonical correlation
analysis
publications:
http://ieeexplore.ieee.org/abstract/document/6993337/
https://link.springer.com/article/10.1007/s10489-017-1012-2
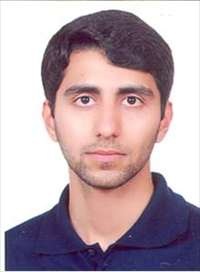